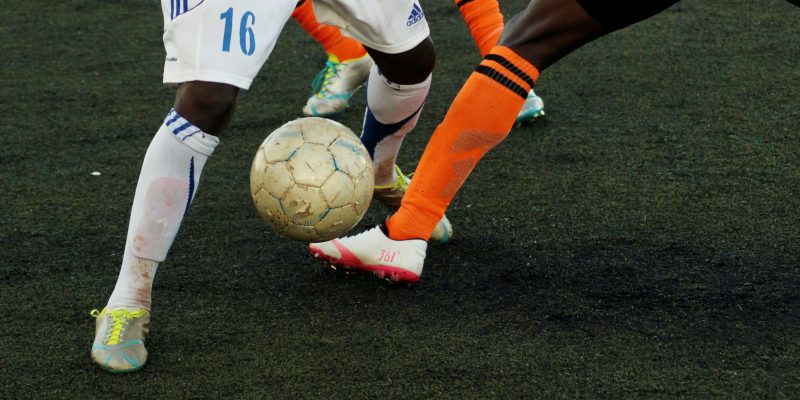
Analyzing Team-Sport Athlete Activity Profiles: A Review
The external burden of a team-sport athlete can be measured using tracking technologies such as global positioning systems (GPS), local positioning systems (LPS), and vision-based systems. These technologies allow for the calculation of displacement, velocity, and acceleration during a match or training session. In order to detect meaningful changes in team-sport athlete external stress, it is critical to precisely quantify these elements.
A brief description
Quantifying athlete external load for training and competition planning and monitoring is of interest to scientists and practitioners. The external load of team-sport athletes can be measured using accelerometers, global positioning systems (GPS), local positioning systems (LPS), and optical tracking systems. Except for accelerometers, these systems calculate displacement, velocity, and acceleration over time. The term “activity profile” refers to an examination of external demand during a game or training session (Aughey, 2011a). The activity profile data is used to track changes over the course of a competitive season or tournament (Bradley et al., 2009; Jennings, D. et al., 2012) and to construct individualised training routines (Bradley et al., 2009). (Boyd et al., 2013).
Athletes who participate in team sports on the field have a well-documented activity profile (Aughey, 2011a; Mooney et al., 2011; Jennings, D. H. et al., 2012; Bradley et al., 2013). In most activity profile analyses, time spent in velocity or acceleration zones is included. Threshold levels arbitrarily chosen by tracking system proprietary software or specified in relation to a physiological test define these zones. There is no consensus on how to calculate a velocity or acceleration threshold at the moment. There are substantial distinctions in the classification of sprint efforts. As a result, it’s difficult to compare activity profiles between and within team sports.
The purpose of this narrative study is to investigate the various velocity and acceleration parameters used to determine external stress in team sports players. When utilising a global velocity or acceleration threshold, individual differences are not taken into account. Physiological tests that include continuous or linear movement do not account for changes in direction or acceleration, despite the fact that particular thresholds can be specified. As a result, current external load analysis approaches are ineffective. Alternative methods, such as unsupervised data mining techniques, are discussed. These approaches can be used to find patterns in external data and to set thresholds.
Athlete Tracking Technologies
External strain on team-sport athletes is measured via tracking technologies. The use of manual video analysis to calculate external load is a low-cost option. Cameras are placed throughout the playing field to film athletes, and the footage is subjectively classified into locomotor categories (Spencer et al., 2004). Manual video analysis takes a long time to complete because it involves observing activity. Validity has not been demonstrated due to the subjective estimation of athlete movement. To detect significant changes in an athlete’s activity profile, a tracking system must be accurate. The ability of a human to accurately duplicate results is a major limitation of manual video analysis. To remove the uncertainty out of athlete activity classification, semi-automated tracking methods were developed. Commercial systems like as ProZone (Di Salvo et al., 2006) and Amisco (Castellano et al., 2014) can detect the position of numerous team-sport athletes. On the other hand, the necessary equipment is both costly and non-portable. As a result, activity profiles cannot be collected without the complicated infrastructure. Athlete movement is also tracked in two dimensions, with vertical movement going unnoticed (Barris and Button, 2008).
Accelerometers are small, wearable sensors that assess three-dimensional athlete load. Accelerometers have been utilised on the field (Mooney et al., 2013) and on the court (Cormack et al., 2014) in team sports, however they cannot define an athlete’s position relative to a playing area. As a result, an athlete’s time and distance travelled at different speeds cannot be measured. In field-based team sports, the use of GPS to calculate distance and velocity is well-documented (Buchheit et al., 2010b; Jennings, D. H. et al., 2012; Varley et al., 2013b). A recent study looked into the factors that determine how GPS data is set up, analysed, and reported for team sports (Malone et al., 2016).